Artificial intelligence (AI) is quickly becoming a vital tool for healthcare providers. AI transforms care delivery from predicting hospital readmissions to streamlining clinical documentation and supporting diagnostic decisions. But with this transformation comes a new wave of complexity — especially for those responsible for rolling out AI initiatives. Data governance, privacy, and compliance all must be considered.
When AI systems interact with sensitive patient data, clinical workflows, or decision-making processes, healthcare leaders must strike a careful balance: How do we accelerate innovation without undermining trust, safety, or regulatory compliance?
The New Challenge: AI Adoption in a Highly Regulated Environment
Hospitals operate under some of the world’s most stringent data privacy and security regulations. The Health Insurance Portability and Accountability Act (HIPAA), Health Information Technology for Economic and Clinical Health (HITECH) Act, and a patchwork of emerging state and federal AI regulations create a complex environment for adopting new technologies.
At the same time, most healthcare data isn’t tidy or uniform. It’s spread across cloud and on-premises systems in formats ranging from structured electronic health records (EHRs) to unstructured physician notes, imaging studies, pathology slides, audio recordings, and genomic data. This reality makes it especially difficult to ensure the integrity and security of data flowing into — and out of — AI systems.
Some of the most common challenges leaders face include:
- Ensuring that AI models only use appropriately secured, authorized datasets
- Maintaining audit trails and explainability around AI-generated outputs
- Addressing bias and fairness concerns in clinical decision support tools
- Governing unstructured data across fragmented environments
- Monitoring for protected health information (PHI) exposure, especially when AI systems interact with third-party tools
Unstructured Data: The Hidden Risk in AI Workflows
While structured data like lab results and billing codes are relatively easy to tag and monitor, unstructured data poses a far greater governance challenge — and it now represents as much as 80% of all healthcare data.
Unstructured data is critical to training AI models, yet it’s also the most likely to contain sensitive patient identifiers or clinical contexts that could be misused if not properly handled. For instance, within Microsoft 365 environments used by many healthcare organizations:
- Microsoft Teams chats and meeting transcripts often include patient updates, clinical handoffs, or informal notes that may reference PHI.
- Outlook emails can contain referral notes, test results, or scheduling details that weren’t entered into the EHR but remain clinically relevant.
- OneDrive documents and Excel files may store ad hoc reports, research notes, or population health analyses that aggregate sensitive data.
- SharePoint folders frequently house draft protocols, care coordination plans, or scanned intake forms uploaded by multiple departments.
Without strong governance, this information can be inadvertently shared, accessed by unauthorized individuals, or pulled into AI systems without proper protection, leading to compliance violations, reputational risk, or even patient harm.
A Governance-First Approach to AI Innovation
To govern unstructured data effectively, healthcare organizations must integrate data classification, access control, and monitoring into their AI strategies from day one:
- Automatically identify and label unstructured data containing PHI or sensitive clinical context.
- Apply role-based access policies that limit how data is used within AI training and inference pipelines.
- Monitor AI data flows in real-time to detect anomalies or policy violations, especially across hybrid and multi-cloud environments.
- Log and audit all AI interactions with unstructured content to ensure transparency, explainability, and compliance readiness.
How AvePoint Supports AI Governance in Healthcare
The AvePoint Confidence Platform is purpose-built to meet these challenges head-on. With AvePoint, healthcare providers can:
- Classify and secure unstructured data across both cloud and on-premises systems — from imaging archives to clinical notes — ensuring sensitive information is properly protected before it enters AI workflows.
- Automate governance policies to enforce HIPAA, Health Information Trust Alliance (HITRUST), and HITECH compliance, reducing manual oversight and risk.
- Gain visibility into AI data pipelines, including where data originates, how it’s accessed, and how it’s used — supporting transparency, auditability, and trust.
- Monitor for real-time policy violations or suspicious data movements, helping teams stay ahead of emerging threats.
- Support responsible AI use by surfacing bias, flagging abnormal AI behavior, and maintaining a complete record of AI-generated decisions.
With AvePoint, healthcare leaders can accelerate AI adoption without compromising compliance or patient trust — bridging the gap between innovation and responsibility. By grounding AI initiatives in robust governance and data security, providers can ensure their efforts truly enhance care delivery while respecting the rights and expectations of patients in a digital age.
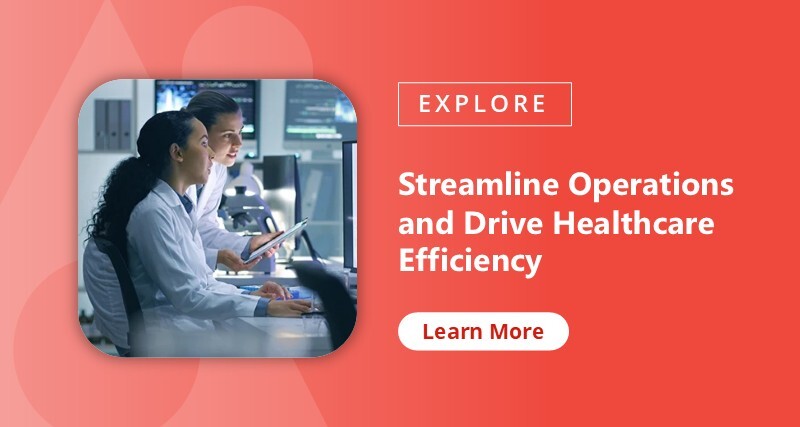